You Can’t Regulate What You Don’t Understand
Or, Why AI Regulations Should Begin with Mandated Disclosures
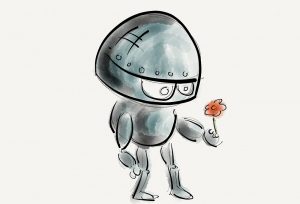
The world changed on November 30, 2022 as surely as it did on August 12, 1908 when the first Model T left the Ford assembly line. That was the date when OpenAI released ChatGPT, the day that AI emerged from research labs into an unsuspecting world. Within two months, ChatGPT had over a hundred million users—faster adoption than any technology in history.
The hand wringing soon began. Most notably, The Future of Life Institute published an open letter calling for an immediate pause in advanced AI research, asking: “Should we let machines flood our information channels with propaganda and untruth? Should we automate away all the jobs, including the fulfilling ones? Should we develop nonhuman minds that might eventually outnumber, outsmart, obsolete and replace us? Should we risk loss of control of our civilization?”
In response, the Association for the Advancement of Artificial Intelligence published its own letter citing the many positive differences that AI is already making in our lives and noting existing efforts to improve AI safety and to understand its impacts. Indeed, there are important ongoing gatherings about AI regulation like the Partnership on AI’s recent convening on Responsible Generative AI, which happened just this past week. The UK has already announced its intention to regulate AI, albeit with a light, “pro-innovation” touch. In the US, Senate Minority Leader Charles Schumer has announced plans to introduce “a framework that outlines a new regulatory regime” for AI. The EU is sure to follow, in the worst case leading to a patchwork of conflicting regulations.
All of these efforts reflect the general consensus that regulations should address issues like data privacy and ownership, bias and fairness, transparency, accountability, and standards. OpenAI’s own AI safety and responsibility guidelines cite those same goals, but in addition call out what many people consider the central, most general question: how do we align AI-based decisions with human values? They write:
“AI systems are becoming a part of everyday life. The key is to ensure that these machines are aligned with human intentions and values.”
But whose human values? Those of the benevolent idealists that most AI critics aspire to be? Those of a public company bound to put shareholder value ahead of customers, suppliers, and society as a whole? Those of criminals or rogue states bent on causing harm to others? Those of someone well meaning who, like Aladdin, expresses an ill-considered wish to an all-powerful AI genie?
There is no simple way to solve the alignment problem. But alignment will be impossible without robust institutions for disclosure and auditing. If we want prosocial outcomes, we need to design and report on the metrics that explicitly aim for those outcomes and measure the extent to which they have been achieved. That is a crucial first step, and we should take it immediately. These systems are still very much under human control. For now, at least, they do what they are told, and when the results don’t match expectations, their training is quickly improved. What we need to know is what they are being told.
What should be disclosed? There is an important lesson for both companies and regulators in the rules by which corporations—which science-fiction writer Charlie Stross has memorably called “slow AIs”—are regulated. One way we hold companies accountable is by requiring them to share their financial results compliant with Generally Accepted Accounting Principles or the International Financial Reporting Standards. If every company had a different way of reporting its finances, it would be impossible to regulate them.
Today, we have dozens of organizations that publish AI principles, but they provide little detailed guidance. They all say things like “Maintain user privacy” and “Avoid unfair bias” but they don’t say exactly under what circumstances companies gather facial images from surveillance cameras, and what they do if there is a disparity in accuracy by skin color. Today, when disclosures happen, they are haphazard and inconsistent, sometimes appearing in research papers, sometimes in earnings calls, and sometimes from whistleblowers. It is almost impossible to compare what is being done now with what was done in the past or what might be done in the future. Companies cite user privacy concerns, trade secrets, the complexity of the system, and various other reasons for limiting disclosures. Instead, they provide only general assurances about their commitment to safe and responsible AI. This is unacceptable.
Imagine, for a moment, if the standards that guide financial reporting simply said that companies must accurately reflect their true financial condition without specifying in detail what that reporting must cover and what “true financial condition” means. Instead, independent standards bodies such as the Financial Accounting Standards Board, which created and oversees GAAP, specify those things in excruciating detail. Regulatory agencies such as the Securities and Exchange Commission then require public companies to file reports according to GAAP, and auditing firms are hired to review and attest to the accuracy of those reports.
So too with AI safety. What we need is something equivalent to GAAP for AI and algorithmic systems more generally. Might we call it the Generally Accepted AI Principles? We need an independent standards body to oversee the standards, regulatory agencies equivalent to the SEC and ESMA to enforce them, and an ecosystem of auditors that is empowered to dig in and make sure that companies and their products are making accurate disclosures.
But if we are to create GAAP for AI, there is a lesson to be learned from the evolution of GAAP itself. The systems of accounting that we take for granted today and use to hold companies accountable were originally developed by medieval merchants for their own use. They were not imposed from without, but were adopted because they allowed merchants to track and manage their own trading ventures. They are universally used by businesses today for the same reason.
So, what better place to start with developing regulations for AI than with the management and control frameworks used by the companies that are developing and deploying advanced AI systems?
The creators of generative AI systems and Large Language Models already have tools for monitoring, modifying, and optimizing them. Techniques such as RLHF (“Reinforcement Learning from Human Feedback”) are used to train models to avoid bias, hate speech, and other forms of bad behavior. The companies are collecting massive amounts of data on how people use these systems. And they are stress testing and “red teaming” them to uncover vulnerabilities. They are post-processing the output, building safety layers, and have begun to harden their systems against “adversarial prompting” and other attempts to subvert the controls they have put in place. But exactly how this stress testing, post processing, and hardening works—or doesn’t—is mostly invisible to regulators.
Regulators should start by formalizing and requiring detailed disclosure about the measurement and control methods already used by those developing and operating advanced AI systems.
In the absence of operational detail from those who actually create and manage advanced AI systems, we run the risk that regulators and advocacy groups “hallucinate” much like Large Language Models do, and fill the gaps in their knowledge with seemingly plausible but impractical ideas.
Companies creating advanced AI should work together to formulate a comprehensive set of operating metrics that can be reported regularly and consistently to regulators and the public, as well as a process for updating those metrics as new best practices emerge.
What we need is an ongoing process by which the creators of AI models fully, regularly, and consistently disclose the metrics that they themselves use to manage and improve their services and to prohibit misuse. Then, as best practices are developed, we need regulators to formalize and require them, much as accounting regulations have formalized the tools that companies already used to manage, control, and improve their finances. It’s not always comfortable to disclose your numbers, but mandated disclosures have proven to be a powerful tool for making sure that companies are actually following best practices.
It is in the interests of the companies developing advanced AI to disclose the methods by which they control AI and the metrics they use to measure success, and to work with their peers on standards for this disclosure. Like the regular financial reporting required of corporations, this reporting must be regular and consistent. But unlike financial disclosures, which are generally mandated only for publicly traded companies, we likely need AI disclosure requirements to apply to much smaller companies as well.
Disclosures should not be limited to the quarterly and annual reports required in finance. For example, AI safety researcher Heather Frase has argued that “a public ledger should be created to report incidents arising from large language models, similar to cyber security or consumer fraud reporting systems.” There should also be dynamic information sharing such as is found in anti-spam systems.
It might also be worthwhile to enable testing by an outside lab to confirm that best practices are being met and what to do when they are not. One interesting historical parallel for product testing may be found in the certification of fire safety and electrical devices by an outside non-profit auditor, Underwriter’s Laboratory. UL certification is not required, but it is widely adopted because it increases consumer trust.
This is not to say that there may not be regulatory imperatives for cutting-edge AI technologies that are outside the existing management frameworks for these systems. Some systems and use cases are riskier than others. National security considerations are a good example. Especially with small LLMs that can be run on a laptop, there is a risk of an irreversible and uncontrollable proliferation of technologies that are still poorly understood. This is what Jeff Bezos has referred to as a “one way door,” a decision that, once made, is very hard to undo. One way decisions require far deeper consideration, and may require regulation from without that runs ahead of existing industry practices.
Furthermore, as Peter Norvig of the Stanford Institute for Human Centered AI noted in a review of a draft of this piece, “We think of ‘Human-Centered AI’ as having three spheres: the user (e.g., for a release-on-bail recommendation system, the user is the judge); the stakeholders (e.g., the accused and their family, plus the victim and family of past or potential future crime); the society at large (e.g. as affected by mass incarceration).”
Princeton computer science professor Arvind Narayanan has noted that these systemic harms to society that transcend the harms to individuals require a much longer term view and broader schemes of measurement than those typically carried out inside corporations. But despite the prognostications of groups such as the Future of Life Institute, which penned the AI Pause letter, it is usually difficult to anticipate these harms in advance. Would an “assembly line pause” in 1908 have led us to anticipate the massive social changes that 20th century industrial production was about to unleash on the world? Would such a pause have made us better or worse off?
Given the radical uncertainty about the progress and impact of AI, we are better served by mandating transparency and building institutions for enforcing accountability than we are in trying to head off every imagined particular harm.
We shouldn’t wait to regulate these systems until they have run amok. But nor should regulators overreact to AI alarmism in the press. Regulations should first focus on disclosure of current monitoring and best practices. In that way, companies, regulators, and guardians of the public interest can learn together how these systems work, how best they can be managed, and what the systemic risks really might be.